NEON Data Science Challenge: Identifying Trees Using Remote Sensing Data
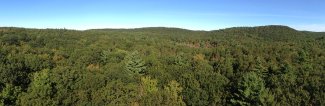
The view of Harvard Forest from the top of NEON's HARV meteorological/flux tower. Massachusetts.
December 2017
What’s the fastest way to count and identify individual trees in a landscape? Using remote sensing data and advanced analytics could help researchers gain new insights into how patterns in tree coverage and growth are changing over time. A team at the University of Florida is challenging the data science community to leverage NEON’s remote sensing data in the NIST-DSE Plant Identification with NEON Remote Sensing Data Challenge.
The challenge is sponsored by the National Institute of Standards and Technology (NIST) Data Science Evaluation (DSE) Series and the Gordon and Betty Moore Foundation’s Data-Driven Discovery Initiative. It was organized by the Data Science Research lab, the WEecology lab, and Stephanie Bohlman’s lab at the University of Florida in active collaboration with NEON. The competition is made possible because of NEON’s airborne data, which is openly available to all researchers.
Participants will utilize data gathered from NEON’s Airborne Observation Platforms. Using sophisticated sensors mounted on low-flying aircraft, NEON collects numerous physical, biological and biochemical data points during annual flights over NEON field sites. The competition challenges data scientists to use this data to accurately classify, measure, and count trees on the ground.
The data points available to contest entrants include:
- Airborne remote sensing: High-resolution hyperspectral imagery, high tecoloresolution RGB imagery, and LiDAR data on plant height.
- Ground Data: Ground-based measurements of tree size, location and type.
- Individual Tree Crowns: Ground-based identification of tree crowns on remote sensing imagery.
Using this data, entrants will need to perform the following tasks:
- Crown Delineation: Estimate the size, shape and location of individual tree crowns.
- Alignment: Pair trees measured on the ground with those identified in remote sensing.
- Classification: Determine the species identity of each tree from remotely sensed data.
Using remote sensing data and data science methods could significantly reduce the time and costs of ecological research related to tree growth, range and biodiversity. Traditional on-the-ground observational data collection is time consuming and expensive, requiring extensive time and labor in the field. Remote sensing data could enable faster data collection and studies of much larger areas than are possible through field studies. However, in order to be useful, the data must be able to be used to accurately determine species, size, and location. The data science challenge uses both field-based and remote sensing data in order to validate the methods used to interpret the data from the airborne remote sensing platforms and demonstrate their utility to answer key research questions.
The competition is open to all, and can be entered by either individuals or teams. More information on the NIST-DSE Plant Identification with NEON Remote Sensing Data challenge can be found at www.ecodse.org. Entries must be received by December 15.
This year’s data science challenge is a pilot. The University of Florida team plans to work with NEON to run an expanded version of the challenge in 2018.